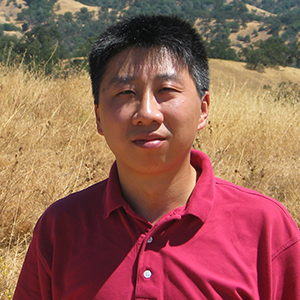
Bio: Joe Lu is a senior manager of data science at Lam Research, a semiconductor wafer fabrication equipment and service provider. He obtained his Ph.D. in computational astrophysics from University of Massachusetts Amherst, and worked as a postdoctoral researcher at Stanford University and Carnegie Institution for Science before joining Lam. In astrophysics, his research was on gaining insight into the formation of galaxies in the Universe from observational data and numerical simulation models. At Lam Research, his work focuses on improving semiconductor equipment and process control by using statistical learning models to gain high precision control over the formation of semiconductor transistors on silicon wafers in plasma chambers.
Abstract: One of the bottlenecks to building semiconductor chips is the increasing cost required to develop chemical plasma processes that form the transistors and memory storage cells. These processes are still developed manually using highly trained engineers searching for a combination of tool parameters that produces an acceptable result on the silicon wafer. The challenge for computer algorithms is the availability of limited experimental data owing to the high cost of acquisition, making it difficult to form a predictive model with accuracy to the atomic scale. In a recent paper we published in Nature (https://doi.org/10.1038/s41586-023-05773-7), we study Bayesian optimization algorithms to investigate how artificial intelligence (AI) might decrease the cost of developing complex semiconductor chip processes. In particular, we create a controlled virtual process game to systematically benchmark the performance of humans and computers for the design of a semiconductor fabrication process. We find that human engineers excel in the early stages of development, whereas the algorithms are far more cost-efficient near the tight tolerances of the target. Furthermore, we show that a strategy using both human designers with high expertise and algorithms in a human first–computer last strategy can reduce the cost-to-target by half compared with only human designers. We will also discuss some cultural challenges in partnering humans with computers that need to be addressed when introducing artificial intelligence in developing semiconductor processes.