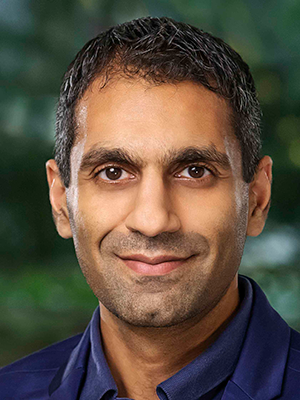
Bio: Naveen Verma received the B.A.Sc. degree in Electrical and Computer Engineering from the UBC, Vancouver, Canada in 2003, and the M.S. and Ph.D. degrees in Electrical Engineering from MIT in 2005 and 2009 respectively. Since July 2009 he has been at Princeton University, where he is current Director of the Keller Center for Innovation in Engineering Education and Professor of Electrical and Computer Engineering. His research focuses on advanced sensing systems, exploring how systems for learning, inference, and action planning can be enhanced by algorithms that exploit new sensing and computing technologies. Prof. Verma co-founded EnCharge AI, together with industry leaders, to commercialize foundational technology for AI computation developed in his lab. Prof. Verma has served as a Distinguished Lecturer of the IEEE Solid-State Circuits Society, and on a number of conference program committees and advisory groups. Finally, he is the recipient of numerous teaching and research awards, including several best-paper awards, with his students.
Abstract: Future data-intensive workloads, particularly from artificial intelligence, have pushed conventional computing architectures to their limits of energy efficiency and throughput, due to the scale of both computations and data they involve. In- and near-memory computing are break through paradigms that provide approaches for overcoming this. But, in doing so, they instate new fundamental tradeoffs that span the device, circuit, and architectural levels. This presentation starts by describing the methods by which in/near-memory computing derive their gains, and then examines the critical tradeoffs, looking concretely at recent designs across memory technologies (SRAM, RRAM, MRAM). Then, its focus turns to key architectural considerations, and how these are likely to drive future technological needs and application alignments. Finally, this presentation analyzes the potential for leveraging application-level relaxations (e.g., noise sensitivity) through algorithmic approaches.