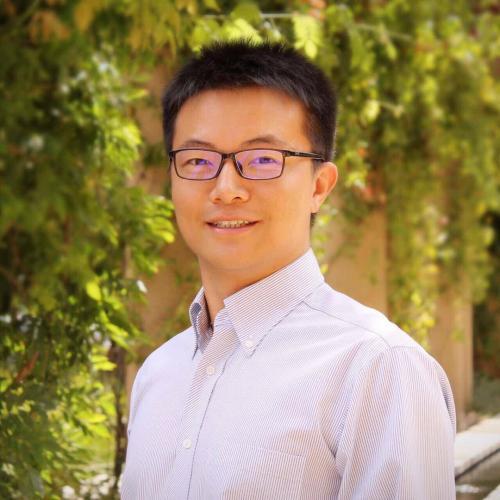
Song’s PhD research pioneered “Deep Compression” as a technique to significantly reduce the storage requirements of deep learning models, without hurting their prediction accuracy. In addition, he developed the “Efficient Inference Engine”, a hardware accelerator which significantly saves memory bandwidth. Finally, he also demonstrated dense-sparse-dense training method to improve the prediction accuracy of deep neural networks. With his research, Song won best-paper awards at the International Conference on Learning Representations and the International Symposium on Field-Programmable Gate Arrays.
The deep compression techniques that Song developed in his PhD have already been adopted by industry. Commercialization of deep compression and other techniques for the optimization of neural networks was also the basis of a start-up founded by Song and colleagues, named DeePhi Tech. DeePhi Tech was just recently acquired by FPGA-leader Xilinx. Song’s PhD research has been featured by O’Reilly, TechEmergence, and The Next Platform, among others.
At MIT, Song is launching a research program centered around the co-design of efficient algorithms and efficient hardware architectures for machine learning with emphasis on their use in resource-constrained mobile systems. In conventional neural networks, the architecture of the network is fixed before training starts. Thus, training cannot improve the architecture. This is very different from how the human brain learns where the neurons and synapses can dynamically prune and grow as learning takes place. Song will investigate “reinforcement learning” approaches for machine learning that will simultaneously optimize the architecture, as well as the model that runs on it.

Co-design of efficient algorithms and hardware architecture for machine learning.