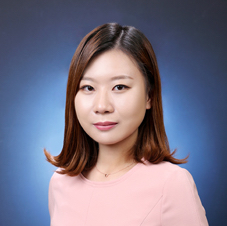
Bio: Kyungmi Lee received a Ph.D. degree in Electrical Engineering and Computer Science from MIT in May 2024. She is currently a Postdoctoral Associate at Prof. Anantha Chandrakasan’s group. Her research interests are security and energy-efficiency of machine learning hardware systems.
Abstract: The security vulnerabilities of off-chip memory can undermine the confidentiality and integrity necessary for deep neural network (DNN) accelerators that process private data and make critical decisions. This work illustrates such vulnerabilities and proposes an effective, hardware-friendly defense solution that provides memory security for DNN accelerators.