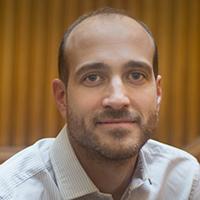
Deep neural networks (DNNs) have quickly become an essential workload across computing form factors, including IoT, mobile, automotive and datacenter. However, DNN inference demands an enormous number of arithmetic operations and a large memory footprint. In this talk, we will explore the co-design of DNN models and hardware to achieve state-of-the-art performance for real-time, energy-constrained inference applications.
This content is restricted to our MIG members and members of the MIT community. Please login or contact us for more information about our partner programs.