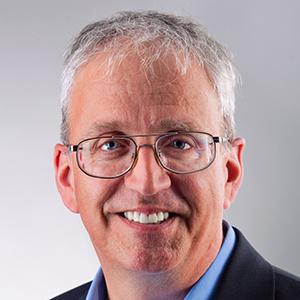
Deep Neural Networks (DNNs) have come to dominate application areas including speech recognition, image understanding, and natural language processing. Most of the technology of DNNs was developed by 1990. However, they were not widely applied until after 2010 when large data sets and powerful GPUs for training became available. These networks place heavy demands on computing hardware for both training and inference. GPUs are ideally suited to training DNNs because of their high floating-point efficiency and memory bandwidth. Efficient communication is essential to scale training across multiple GPUs. For inference, hardware accelerators can offer advantages particularly on sparse and compressed networks. This talk will examine the current state of the art in hardware for deep learning.
This content is restricted to our MIG members and members of the MIT community. Please login or contact us for more information about our partner programs.